Estimación del área de playa a través de información satelital de libre acceso: calibración para la costa de Montevideo, Uruguay
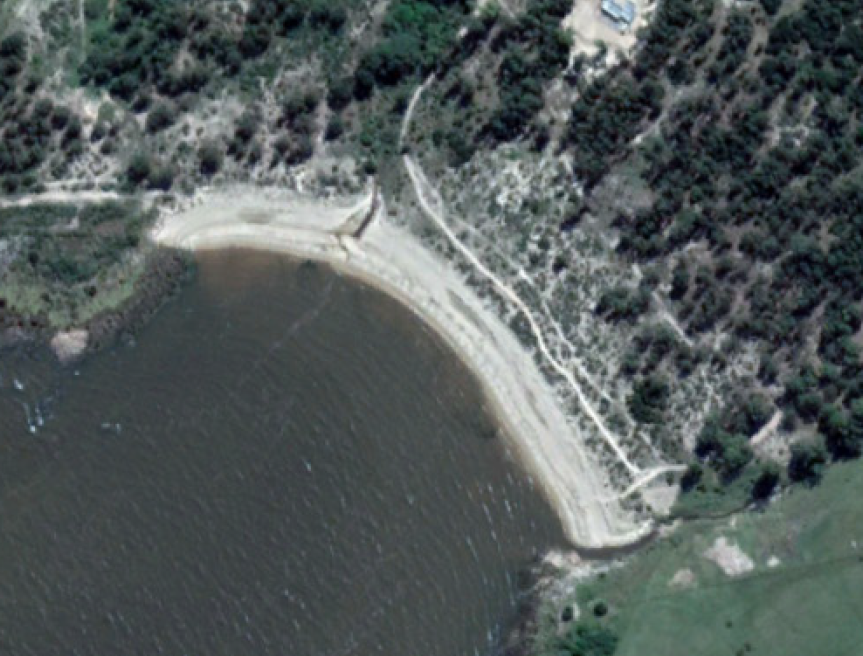
Publicado 2023-06-15
Palabras clave
- manejo ecosistémico,
- Landsat,
- acceso libre,
- playas arenosas,
- erosión costera
Cómo citar
Derechos de autor 2023 Luis Orlando

Esta obra está bajo una licencia internacional Creative Commons Atribución-NoComercial-CompartirIgual 4.0.
Resumen
Las playas arenosas brindan gran variedad de servicios ecosistémicos sosteniendo el bienestar humano en áreas costeras. Estos
ecosistemas dinámicos dependen de la interacción entre: el oleaje, las mareas y los regímenes de viento. Su alta variabilidad los hace
vulnerables a las modificaciones físicas y el cambio climático, amenazando la estabilidad de las funciones ecosistémicas. El análisis
de la dinámica costera requiere una gran cantidad de información, este estudio utiliza una metodología de acceso libre para estimar el área de playa a través de imágenes satelitales de la colección Landsat. El área de las playas es una variable relevante para dilucidar la dinámica costera, los atributos ecosistémicos y el potencial turístico. La arena (mediante Random Forest) y la cobertura vegetal (mediante el índice de diferencias normalizadas de vegetación) se consideraron como componentes del ecosistema de playa. Se validó el método para la costa de Montevideo, comparando los resultados con medidas independientes, y se estimó el área de 20 playas de para un período de 35 años. Esta metodología está disponible para su aplicación a un costo operativo bajo, representado una oportunidad para aumentar la información disponible y mejorar el manejo en materia de dinámica costera y uso de las playas arenosas.
Descargas
Citas
- Amyot, J. and J. Grant. 2014. Environmental function analysis: A decision support tool for integrated sandy beach planning. Ocean Coast Manag, 102: 317–327. https://doi.org/10.1016/j.ocecoaman.2014.10.009
- Barnard, P.L., D.M. Hubbard and J.E. Dugan. 2012. Beach response dynamics of a littoral cell using a 17-year single-point time series of sand thickness. Geomorphology 140: 588–598. https://doi.org/10.1016/j.geomorph.2011.12.023
- Barnard, P.L., A.D. Short, M.D. Harley, K.D. Splinter, S. Vitousek, I.L. Turner, J. Allan, M. Banno, K.R. Bryan, A. Doria, J.E. Hansen, S. Kato, Y. Kuriyama, P. Randall-Goodwin, P. Ruggiero, I.J. Walker and D.K. Heathfield. 2015. Coastal vulnerability across the Pacific dominated by El Niño/Southern Oscillation. Nat. Geosci., 8: 801.
- Boak, E. and I. Turner. 2005. Shoreline definition and detection: A review. J. Coast. Res., 21: 688–703. https://doi.org/10.2112/03-0071.1
- Breiman, L. 2001. Random forests. Mach Learn, 45: 5–32.
- Brieuc, M.S.O., C.D. Waters, D.P. Drinan and K.A. Naish. 2018. A practical introduction to Random Forest for genetic association studies in ecology and evolution. Mol. Ecol. Resour., 18(4): 755–766. https://doi.org/10.1111/1755-0998.12773
- César de Sá, N., S. Carvalho, P. Castro, E., Marchante and H. Marchante. 2017. Using Landsat time series to understand how management and disturbances influence the expansion of an invasive tree. IEEE J. Sel. Topics App. Earth Obs. Rem. Sens., 10(7): 3243–3253.
- Cifuentes Ossa, M.A., L.V. Rosero Henao y J.J. Selvaraj. 2017. Detección de cambios de la línea costera al norte del distrito de Buenaventura mediante el uso de sensores remotos. Bol. Invest. Mar. Costeras, 46. https://doi.org/10.25268/bimc.invemar.2017.46.1.719
- Defeo, O. and M. Elliott. 2020. The ‘triple whammy’ of coasts under threat – Why we should be worried! Mar. Pollut. Bull., 111832. https://doi.org/10.1016/j.marpolbul.2020.111832
- Defeo, O., A. McLachlan, D.S. Schoeman, T.A. Schlacher, J. Dugan, A. Jones, M. Lastra and F. Scapini. 2009. Threats to sandy beach ecosystems: A review. Estuar. Coast. Shelf Sci., 81: 1–12. https://doi.org/10.1016/j.ecss.2008.09.022
- García-Alonso, J., D. Lercari, B.F. Araujo, M.G. Almeida and C.E. Rezende.2017. Total and extractable elemental composition of the intertidal estuarine biofilm of the Río de la Plata: Disentangling natural and anthropogenic influences. Estuar. Coast. Shelf Sci., 187: 53–61. https://doi.org/10.1016/j.ecss.2016.12.018
- Gao, B. 1996. NDWI—A normalized difference water index for remote sensing of vegetation liquid water from space. Rem. Sens. Environ., 58: 257–266. https://doi.org/10.1016/S0034-4257(96)00067-3
- Gorelick, N., M. Hancher, M. Dixon, S. Ilyushchenko, D. Thau and R. Moore. 2017. Google Earth Engine: Planetary-scale geospatial analysis for everyone. Rem. Sens. Environ., 202: 18–27. https://doi.org/10.1016/j.rse.2017.06.031
- Gutiérrez, O. 2010. Dinámica sedimentaria en la costa uruguaya: evolución y tendencias de playas urbanas en el marco del Cambio Global. Tesis Magíster Ciencias Ambientales, UdelaR, Montevideo. 98 p. https://doi.org/10.13140/RG.2.1.1904.4568
- Gutiérrez, O., D. Panario, G.J. Nagy, G. Piñeiro and C. Montes. 2015. Long-term morphological evolution of urban pocket beaches in Montevideo (Uruguay): Impacts of coastal interventions and links to climate forcing. J. Integr. Coast. Zone Manag., 15: 467-484. https://doi.org/10.5894/rgci553
- Gutiérrez, O., D. Panario, G.J. Nagy, M. Bidegain and C. Montes. 2016. Climate teleconnections and indicators of coastal systems response. Ocean Coast. Manag., 122: 64–76. https://doi.org/10.1016/j.ocecoaman.2016.01.009
- Harley, M.D., I.L Turner, A.D. Short and R. Ranasinghe. 2010. Assessment and integration of conventional, RTK-GPS and image-derived beach survey methods for daily to decadal coastal monitoring. Coast. Eng, 58: 194–205. https://doi.org/10.1016/j.coastaleng.2010.09.006
- Harris, L., R. Nel and D. Schoeman. 2011. Mapping beach morphodynamics remotely: A novel application tested on South African sandy shores. Estuar. Coast. Shelf Sci., 92: 78–89. https://doi.org/10.1016/j.ecss.2010.12.013
- Jensen, J.R. 2005. Introductory digital image processing: A remote sensing perspective. Prentice Hall, Upper Saddle River. 512 p.
- Lercari, D. and O. Defeo. 2015. Large-scale dynamics of sandy beach ecosystems in transitional waters of the southwestern Atlantic Ocean: Species turnover, stability and spatial synchrony. Estuar. Coast. Shelf Sci., 154: 184–193. https://doi.org/10.1016/j.ecss.2015.01.011
- Luijendijk, A., G. Hagenaars, R. Ranasinghe, F. Baart, G. Donchyts and S. Aarninkhof. 2018. The state of the world’s beaches. Sci. Rep., 8: 6641. https://doi.org/10.1038/s41598-018-24630-6
- McLachlan, A. and O. Defeo. 2018. The ecology of sandy shores. Academic Press, London. 542 p. https://doi.org/10.1016/B978-0-12-809467-9.00001-1
- McLachlan, A., O. Defeo, E. Jaramillo and A.D. Short. 2013. Sandy beach conservation and recreation: Guidelines for optimizing management strategies for multi-purpose use. Ocean Coast. Manag., 71: 256–268. https://doi.org/10.1016/j.ocecoaman.2012.10.005
- McLachlan, A., O. Defeo and A.D. Short. 2018. Characterizing sandy beaches into major types and states: Implications for ecologists and managers. Estuar. Coast. Shelf Sci., 215: 152–160. https://doi.org/10.1016/j.ecss.2018.09.027
- Millard, K. and M. Richardson. 2015. On the importance of training data sample selection in Random Forest image classification: A case study in peatland ecosystem mapping. Rem. Sens., 7: 8489–8515.
- Orlando, L. 2020. BeachAreaMontevideo1984_2019 [Data set]. Zenodo. http://doi.org/10.5281/zenodo.4327667
- Orlando, L., L. Ortega and O. Defeo. 2019. Multi-decadal variability in sandy beach area and the role of climate forcing. Estuar. Coast. Shelf Sci., 218: 197–203. https://doi.org/10.1016/j.ecss.2018.12.015
- Orlando, L., L. Ortega and O. Defeo. 2020. Urbanization effects on sandy beach macrofauna along an estuarine gradient. Ecol. Ind., 111: 106036. https://doi.org/10.1016/j.ecolind.2019.106036
- Orlando, L., L. Ortega and O. Defeo. 2021. Perspectives for sandy beach management in the Anthropocene: Satellite information, tourism seasonality, and expert recommendations. Estuar. Coast. Shelf Sci., 107597. https://doi.org/10.1016/j.ecss.2021.107597
- Ozturk, D. and F. Sesli. 2015. Shoreline change analysis of the Kizilirmak Lagoon series. Ocean Coast. Manag., 118: 290–308. https://doi.org/10.1016/j.ocecoaman.2015.03.009
- R Development Core Team. 2013. R: A language and environment for statistical computing. R Foundation for Statistical Computing. Vienna, Austria. https://cran.r-project.org/ 8/09/2020.
- Sepúlveda, H.H., A. Valle-Levinson and M.B. Framiñán. 2004. Observations of subtidal and tidal flow in the Rı́o de la Plata Estuary. Cont. Shelf Res., 24:509–525. https://doi.org/10.1016/j.csr.2003.12.002
- Short, A.D. 1999. Beach and shoreface morphodynamics. Wiley, Chichester. 379 p.
- Short, A.D. and D.W.T. Jackson. 2013. Beach morphodynamics: 106-129. In: Treatise on Geomorphology. Academic Press, San Diego.
- Simionato, C.G., M.L. Clara Tejedor, C. Campetella, R. Guerrero and D. Moreira. 2010. Patterns of sea surface temperature variability on seasonal to subannual scales at and offshore the Río de la Plata estuary. Cont. Shelf Res., 30: 1983–1997. https://doi.org/10.1016/j.csr.2010.09.012
- Splinter, K.D., I.L. Turner and M.A. Davidson. 2013. How much data is enough? The importance of morphological sampling interval and duration for calibration of empirical shoreline models. Coast. Eng., 77: 14–27. https://doi.org/10.1016/j.coastaleng.2013.02.009
- Takimoto, G. and D.M. Post. 2013. Environmental determinants of food-chain length: a meta-analysis. Ecol. Res., 28: 675–681. https://doi.org/10.1007/s11284-012-0943-7
- Verocai, J.E., M. Gómez-Erache, G.J. Nagy and M. Bidegain. 2015. Addressing climate extremes in Coastal Management: The case of the Uruguayan coast of the Rio de la Plata System. J. Integr. Coast. Zone Manag., 15:91-107. https://doi.org/10.5894/rgci555
- Vos, K., M.D. Harley, K.D. Splinter, J.A. Simmons and I.L. Turner. 2019. Sub-annual to multi-decadal shoreline variability from publicly available satellite imagery. Coast. Eng., 150: 160–174. https://doi.org/10.1016/j.coastaleng.2019.04.004